PI: Steven Bradfute & Christophe Lambert
Duration: 11/2022 – 11/ 2027
Goal: Develop unsupervised Machine Learning and Artificial Intelligence to select antivirals against alphavirals
Alphaviruses are biothreat agents for which there are no approved vaccines or cure. Traditional drug screening for antiviral compounds has been plagued by a high rate of in vitro success followed by in vivo failure, due to lack of efficacy, off-target activity, or unfavorable toxicity/bioavailability characteristics. Screening costs can also prohibit large-scale testing for efficacy against viral infections, especially against high-containment pathogens. Here we propose to combine innovations in non-negative matrix factorization (NMF), semi-supervised positive-unlabeled Machine Learning/Artificial Intelligence (ML/AI), coupled with an iterative wet-lab experimental testing and feedback loop, to identify novel antivirals with favorable absorption, distribution, metabolism, excretion, and toxicity (ADMET) properties that target encephalitic alphaviral proteins involved in the viral life cycle. Our approach is unique in the combined use of novel unsupervised and semi-supervised ML/AI informed by multiple rounds of in vitro testing using live BSL-3 alphaviruses to improve both ML/AI model performance and in vivo efficacy
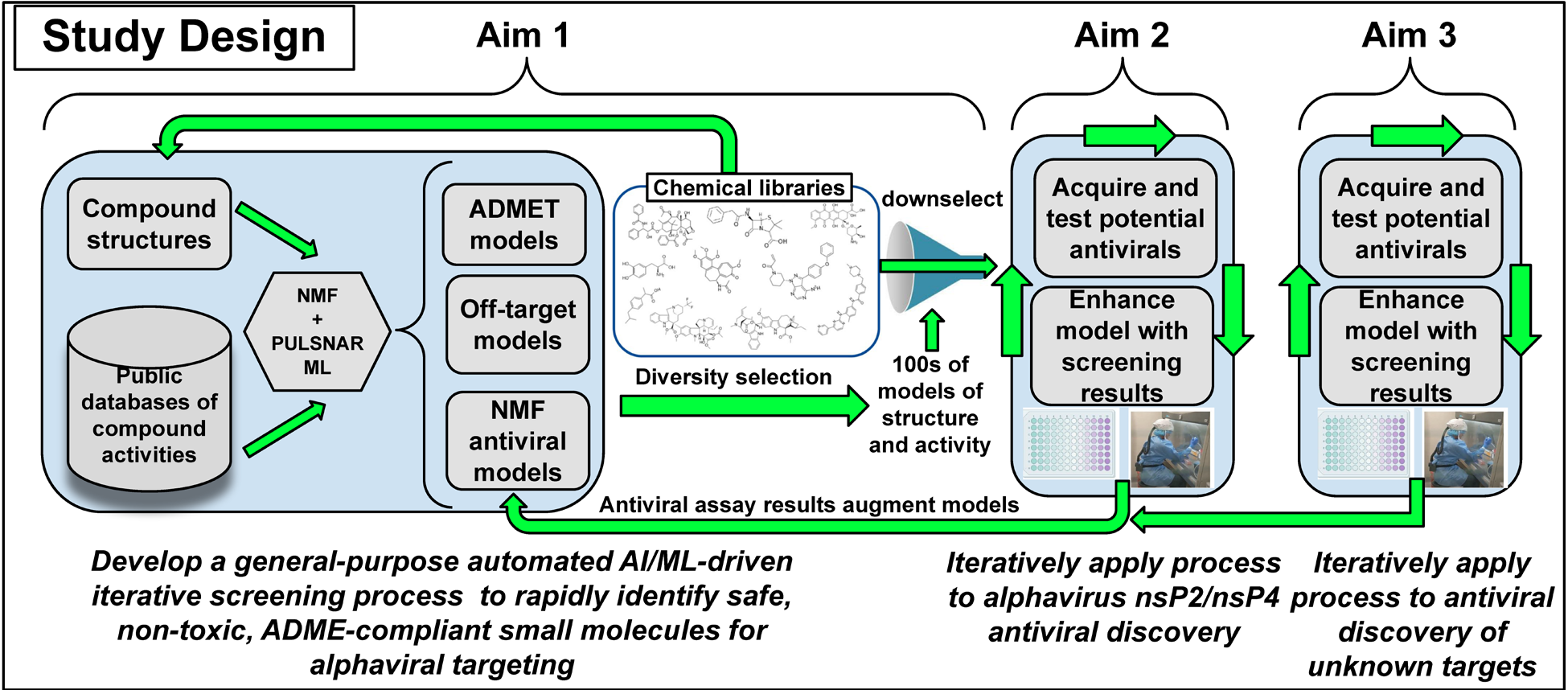